10.8: t Distribution
( \newcommand{\kernel}{\mathrm{null}\,}\)
Learning Objectives
- State the difference between the shape of the t distribution and the normal distribution
- State how the difference between the shape of the t distribution and normal distribution is affected by the degrees of freedom
- Use a t table to find the value of t to use in a confidence interval
- Use the t calculator to find the value of t to use in a confidence interval
In the introduction to normal distributions it was shown that 95% of the area of a normal distribution is within 1.96 standard deviations of the mean. Therefore, if you randomly sampled a value from a normal distribution with a mean of 100, the probability it would be within 1.96σ of 100 is 0.95. Similarly, if you sample N values from the population, the probability that the sample mean (M) will be within 1.96σM of 100 is 0.95.
Now consider the case in which you have a normal distribution but you do not know the standard deviation. You sample N values and compute the sample mean (M) and estimate the standard error of the mean (σM) with sM. What is the probability that M will be within 1.96sM of the population mean (μ)?
This is a difficult problem because there are two ways in which M could be more than 1.96sM from μ:
- M could, by chance, be either very high or very low and
- sM could, by chance, be very low.
Intuitively, it makes sense that the probability of being within 1.96 standard errors of the mean should be smaller than in the case when the standard deviation is known (and cannot be underestimated). But exactly how much smaller? Fortunately, the way to work out this type of problem was solved in the early 20th century by W. S. Gosset who determined the distribution of a mean divided by an estimate of its standard error. This distribution is called the Student's t distribution or sometimes just the t distribution. Gosset worked out the t distribution and associated statistical tests while working for a brewery in Ireland. Because of a contractual agreement with the brewery, he published the article under the pseudonym "Student." That is why the t test is called the "Student's t test."
The t distribution is very similar to the normal distribution when the estimate of variance is based on many degrees of freedom, but has relatively more scores in its tails when there are fewer degrees of freedom. Figure 10.8.1 shows t distributions with 2, 4, and 10 degrees of freedom and the standard normal distribution. Notice that the normal distribution has relatively more scores in the center of the distribution and the t distribution has relatively more in the tails. The t distribution is therefore leptokurtic. The t distribution approaches the normal distribution as the degrees of freedom increase.
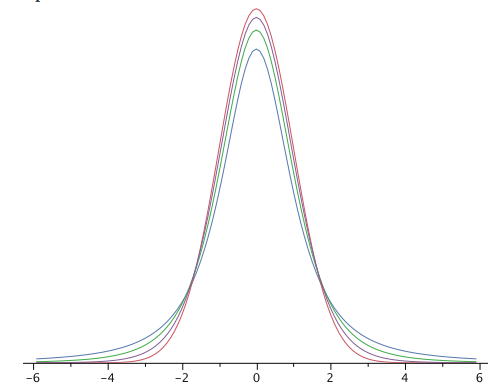
Since the t distribution is leptokurtic, the percentage of the distribution within 1.96 standard deviations of the mean is less than the 95% for the normal distribution. Table 10.8.1 shows the number of standard deviations from the mean required to contain 95% and 99% of the area of the t distribution for various degrees of freedom. These are the values of t that you use in a confidence interval. The corresponding values for the normal distribution are 1.96 and 2.58 respectively. Notice that with few degrees of freedom, the values of t are much higher than the corresponding values for a normal distribution and that the difference decreases as the degrees of freedom increase. The values in Table 10.8.1 can be obtained from the "Find t for a confidence interval" calculator.
Table 10.8.1: Abbreviated t table
df | 0.95 | 0.99 |
---|---|---|
2 | 4.303 | 9.925 |
3 | 3.182 | 5.841 |
4 | 2.776 | 4.604 |
5 | 2.571 | 4.032 |
8 | 2.306 | 3.355 |
10 | 2.228 | 3.169 |
20 | 2.086 | 2.845 |
50 | 2.009 | 2.678 |
100 | 1.984 | 2.626 |
Returning to the problem posed at the beginning of this section, suppose you sampled 9 values from a normal population and estimated the standard error of the mean (σM) with sM. What is the probability that M would be within 1.96sM of μ? Since the sample size is 9, there are N−1=8df. From Table 10.8.1 you can see that with 8df the probability is 0.95 that the mean will be within 2.306sM of μ. The probability that it will be within 1.96sM of μ is therefore lower than 0.95.
As shown in Figure 10.8.2, the "t distribution" calculator can be used to find that 0.086 of the area of a t distribution is more than 1.96 standard deviations from the mean, so the probability that M would be less than 1.96sM from μ is 1−0.086=0.914.
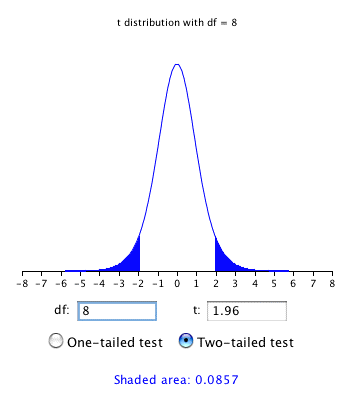
As expected, this probability is less than 0.95 that would have been obtained if σM had been known instead of estimated.