13.7: Lotteries
- Page ID
- 10261
\( \newcommand{\vecs}[1]{\overset { \scriptstyle \rightharpoonup} {\mathbf{#1}} } \)
\( \newcommand{\vecd}[1]{\overset{-\!-\!\rightharpoonup}{\vphantom{a}\smash {#1}}} \)
\( \newcommand{\id}{\mathrm{id}}\) \( \newcommand{\Span}{\mathrm{span}}\)
( \newcommand{\kernel}{\mathrm{null}\,}\) \( \newcommand{\range}{\mathrm{range}\,}\)
\( \newcommand{\RealPart}{\mathrm{Re}}\) \( \newcommand{\ImaginaryPart}{\mathrm{Im}}\)
\( \newcommand{\Argument}{\mathrm{Arg}}\) \( \newcommand{\norm}[1]{\| #1 \|}\)
\( \newcommand{\inner}[2]{\langle #1, #2 \rangle}\)
\( \newcommand{\Span}{\mathrm{span}}\)
\( \newcommand{\id}{\mathrm{id}}\)
\( \newcommand{\Span}{\mathrm{span}}\)
\( \newcommand{\kernel}{\mathrm{null}\,}\)
\( \newcommand{\range}{\mathrm{range}\,}\)
\( \newcommand{\RealPart}{\mathrm{Re}}\)
\( \newcommand{\ImaginaryPart}{\mathrm{Im}}\)
\( \newcommand{\Argument}{\mathrm{Arg}}\)
\( \newcommand{\norm}[1]{\| #1 \|}\)
\( \newcommand{\inner}[2]{\langle #1, #2 \rangle}\)
\( \newcommand{\Span}{\mathrm{span}}\) \( \newcommand{\AA}{\unicode[.8,0]{x212B}}\)
\( \newcommand{\vectorA}[1]{\vec{#1}} % arrow\)
\( \newcommand{\vectorAt}[1]{\vec{\text{#1}}} % arrow\)
\( \newcommand{\vectorB}[1]{\overset { \scriptstyle \rightharpoonup} {\mathbf{#1}} } \)
\( \newcommand{\vectorC}[1]{\textbf{#1}} \)
\( \newcommand{\vectorD}[1]{\overrightarrow{#1}} \)
\( \newcommand{\vectorDt}[1]{\overrightarrow{\text{#1}}} \)
\( \newcommand{\vectE}[1]{\overset{-\!-\!\rightharpoonup}{\vphantom{a}\smash{\mathbf {#1}}}} \)
\( \newcommand{\vecs}[1]{\overset { \scriptstyle \rightharpoonup} {\mathbf{#1}} } \)
\( \newcommand{\vecd}[1]{\overset{-\!-\!\rightharpoonup}{\vphantom{a}\smash {#1}}} \)
\(\newcommand{\avec}{\mathbf a}\) \(\newcommand{\bvec}{\mathbf b}\) \(\newcommand{\cvec}{\mathbf c}\) \(\newcommand{\dvec}{\mathbf d}\) \(\newcommand{\dtil}{\widetilde{\mathbf d}}\) \(\newcommand{\evec}{\mathbf e}\) \(\newcommand{\fvec}{\mathbf f}\) \(\newcommand{\nvec}{\mathbf n}\) \(\newcommand{\pvec}{\mathbf p}\) \(\newcommand{\qvec}{\mathbf q}\) \(\newcommand{\svec}{\mathbf s}\) \(\newcommand{\tvec}{\mathbf t}\) \(\newcommand{\uvec}{\mathbf u}\) \(\newcommand{\vvec}{\mathbf v}\) \(\newcommand{\wvec}{\mathbf w}\) \(\newcommand{\xvec}{\mathbf x}\) \(\newcommand{\yvec}{\mathbf y}\) \(\newcommand{\zvec}{\mathbf z}\) \(\newcommand{\rvec}{\mathbf r}\) \(\newcommand{\mvec}{\mathbf m}\) \(\newcommand{\zerovec}{\mathbf 0}\) \(\newcommand{\onevec}{\mathbf 1}\) \(\newcommand{\real}{\mathbb R}\) \(\newcommand{\twovec}[2]{\left[\begin{array}{r}#1 \\ #2 \end{array}\right]}\) \(\newcommand{\ctwovec}[2]{\left[\begin{array}{c}#1 \\ #2 \end{array}\right]}\) \(\newcommand{\threevec}[3]{\left[\begin{array}{r}#1 \\ #2 \\ #3 \end{array}\right]}\) \(\newcommand{\cthreevec}[3]{\left[\begin{array}{c}#1 \\ #2 \\ #3 \end{array}\right]}\) \(\newcommand{\fourvec}[4]{\left[\begin{array}{r}#1 \\ #2 \\ #3 \\ #4 \end{array}\right]}\) \(\newcommand{\cfourvec}[4]{\left[\begin{array}{c}#1 \\ #2 \\ #3 \\ #4 \end{array}\right]}\) \(\newcommand{\fivevec}[5]{\left[\begin{array}{r}#1 \\ #2 \\ #3 \\ #4 \\ #5 \\ \end{array}\right]}\) \(\newcommand{\cfivevec}[5]{\left[\begin{array}{c}#1 \\ #2 \\ #3 \\ #4 \\ #5 \\ \end{array}\right]}\) \(\newcommand{\mattwo}[4]{\left[\begin{array}{rr}#1 \amp #2 \\ #3 \amp #4 \\ \end{array}\right]}\) \(\newcommand{\laspan}[1]{\text{Span}\{#1\}}\) \(\newcommand{\bcal}{\cal B}\) \(\newcommand{\ccal}{\cal C}\) \(\newcommand{\scal}{\cal S}\) \(\newcommand{\wcal}{\cal W}\) \(\newcommand{\ecal}{\cal E}\) \(\newcommand{\coords}[2]{\left\{#1\right\}_{#2}}\) \(\newcommand{\gray}[1]{\color{gray}{#1}}\) \(\newcommand{\lgray}[1]{\color{lightgray}{#1}}\) \(\newcommand{\rank}{\operatorname{rank}}\) \(\newcommand{\row}{\text{Row}}\) \(\newcommand{\col}{\text{Col}}\) \(\renewcommand{\row}{\text{Row}}\) \(\newcommand{\nul}{\text{Nul}}\) \(\newcommand{\var}{\text{Var}}\) \(\newcommand{\corr}{\text{corr}}\) \(\newcommand{\len}[1]{\left|#1\right|}\) \(\newcommand{\bbar}{\overline{\bvec}}\) \(\newcommand{\bhat}{\widehat{\bvec}}\) \(\newcommand{\bperp}{\bvec^\perp}\) \(\newcommand{\xhat}{\widehat{\xvec}}\) \(\newcommand{\vhat}{\widehat{\vvec}}\) \(\newcommand{\uhat}{\widehat{\uvec}}\) \(\newcommand{\what}{\widehat{\wvec}}\) \(\newcommand{\Sighat}{\widehat{\Sigma}}\) \(\newcommand{\lt}{<}\) \(\newcommand{\gt}{>}\) \(\newcommand{\amp}{&}\) \(\definecolor{fillinmathshade}{gray}{0.9}\)You realize the odds of winning [the lottery] are the same as being mauled by a polar bear and a regular bear in the same day.
—E*TRADE baby, January 2010.
Lotteries are among the simplest and most widely played of all games of chance, and unfortunately for the gambler, among the worst in terms of expected value. Lotteries come in such an incredible number of variations that it is impractical to analyze all of them. So, in this section, we will study some of the more common lottery formats.
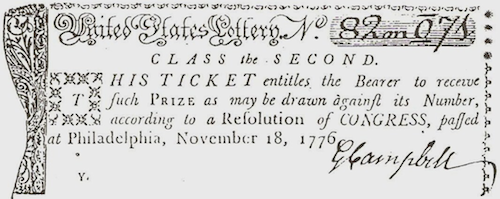
The Basic Lottery
Basic Format
The basic lottery is a random experiment in which the gambling house (in many cases a government agency) selects \(n\) numbers at random, without replacement, from the integers from 1 to \(N\). The integer parameters \(N\) and \(n\) vary from one lottery to another, and of course, \(n\) cannot be larger than \(N\). The order in which the numbers are chosen usually does not matter, and thus in this case, the sample space \(S\) of the experiment consists of all subsets (combinations) of size \(n\) chosen from the population \(\{1, 2, \ldots, N\}\). \[ S = \left\{ \bs{x} \subseteq \{1, 2, \ldots, N\}: \#(\bs{x}) = n\right\} \]
Recall that \[ \#(S) = \binom{N}{n} = \frac{N!}{n! (N - n)!}\]
Naturally, we assume that all such combinations are equally likely, and thus, the chosen combination \(\bs{X}\), the basic random variable of the experiment, is uniformly distributed on \(S\). \[ \P(\bs{X} = \bs{x}) = \frac{1}{\binom{N}{n}}, \quad \bs{x} \in S \] The player of the lottery pays a fee and gets to select \(m\) numbers, without replacement, from the integers from 1 to \(N\). Again, order does not matter, so the player essentially chooses a combination \(\bs{y}\) of size \(m\) from the population \(\{1, 2, \ldots, N\}\). In many cases \(m = n\), so that the player gets to choose the same number of numbers as the house. In general then, there are three parameters in the basic \((N, n, m)\) lottery.
The player's goal, of course, is to maximize the number of matches (often called catches by gamblers) between her combination \(\bs{y}\) and the random combination \(\bs{X}\) chosen by the house. Essentially, the player is trying to guess the outcome of the random experiment before it is run. Thus, let \(U = \#(\bs{X} \cap \bs{y})\) denote the number of catches.
The number of catches \(U\) in the \((N, n, m)\), lottery has probability density function given by \[ \P(U = k) = \frac{\binom{m}{k} \binom{N - m}{n - k}}{\binom{N}{n}}, \quad k \in \{0, 1, \ldots, m\} \]
The distribution of \(U\) is the hypergeometric distribution with parameters \(N\), \(n\), and \(m\), and is studied in detail in the chapter on Finite Sampling Models. In particular, from this section, it follows that the mean and variance of the number of catches \(U\) are \begin{align} \E(U) = & n \frac{m}{N} \\ \var(U) = & n \frac{m}{N} \left(1 - \frac{m}{N}\right) \frac{N - n}{N - 1} \end{align} Note that \(\P(U = k) = 0\) if \(k \gt n\) or \(k \lt n + m - N\). However, in most lotteries, \(m \le n\) and \(N\) is much larger than \(n + m\). In these common cases, the density function is positive for the values of \(k\) given in above.
We will refer to the special case where \(m = n\) as the \((N, n)\) lottery; this is the case in most state lotteries. In this case, the probability density function of the number of catches \(U\) is \[ \P(U = k) = \frac{\binom{n}{k} \binom{N - n}{n - k}}{\binom{N}{n}}, \quad k \in \{0, 1, \ldots, n\} \] The mean and variance of the number of catches \(U\) in this special case are \begin{align} \E(U) & = \frac{n^2}{N} \\ \var(U) & = \frac{n^2 (N - n)^2}{N^2 (N - 1)} \end{align}
Explicitly give the probability density function, mean, and standard deviation of the number of catches in the \((47, 5)\) lottery.
Answer
\(\E(U) = 0.5319148936\), \(\sd(U) = 0.6587832083\)
\(k\) | \(\P(U = k)\) |
---|---|
0 | 0.5545644253 |
1 | 0.3648450167 |
2 | 0.0748400034 |
3 | 0.0056130003 |
4 | 0.0001369024 |
5 | 0.0000006519 |
Explicitly give the probability density function, mean, and standard deviation of the number of catches in the \((49, 5)\) lottery.
Answer
\(\E(U) = 0.5102040816\), \(\sd(U) = 0.6480462207\)
\(k\) | \(\P(U = k)\) |
---|---|
0 | 0.5695196981 |
1 | 0.3559498113 |
2 | 0.0694536217 |
3 | 0.0049609730 |
4 | 0.0001153715 |
5 | 0.0000005244 |
Explicitly give the probability density function, mean, and standard deviation of the number of catches in the \((47, 7)\) lottery.
Answer
\(\E(U) = 1.042553191\), \(\sd(U) = 0.8783776109\)
\(k\) | \(\P(U = k)\) |
---|---|
0 | 0.2964400642 |
1 | 0.4272224454 |
2 | 0.2197144005 |
3 | 0.0508598149 |
4 | 0.0054983583 |
5 | 0.0002604486 |
6 | 0.0000044521 |
7 | 0.0000000159 |
The analysis above was based on the assumption that the player's combination \(\bs{y}\) is selected deterministically. Would it matter if the player chose the combination in a random way? Thus, suppose that the player's selected combination \(\bs{Y}\) is a random variable taking values in \(S\). (For example, in many lotteries, players can buy tickets with combinations randomly selected by a computer; this is typically known as Quick Pick). Clearly, \(\bs{X}\) and \(\bs{Y}\) must be independent, since the player (and her randomizing device) can have no knowledge of the winning combination \(\bs{X}\). As you might guess, such randomization makes no difference.
Let \(U\) denote the number of catches in the \((N, n, m)\) lottery when the player's combination \(\bs{Y}\) is a random variable, independent of the winning combination \(\bs{X}\). Then \(U\) has the same distribution as in the deterministic case above.
Proof
This follows by conditioning on the value of \(\bs{Y}\): \[ \P(U = k) = \sum_{\bs{y} \in S} \P(U = k \mid \bs{Y} = \bs{y}) \P(\bs{Y} = \bs{y}) = \sum_{\bs{y} \in S} \P(U = k) \P(\bs{Y} = \bs{y}) = \P(U = k) \]
There are many websites that publish data on the frequency of occurrence of numbers in various state lotteries. Some gamblers evidently feel that some numbers are luckier than others.
Given the assumptions and analysis above, do you believe that some numbers are luckier than others? Does it make any mathematical sense to study historical data for a lottery?
The prize money in most state lotteries depends on the sales of the lottery tickets. Typically, about 50% of the sales money is returned as prize money; the rest goes for administrative costs and profit for the state. The total prize money is divided among the winning tickets, and the prize for a given ticket depends on the number of catches \(U\). For all of these reasons, it is impossible to give a simple mathematical analysis of the expected value of playing a given state lottery. Note however, that since the state keeps a fixed percentage of the sales, there is essentially no risk for the state.
From a pure gambling point of view, state lotteries are bad games. In most casino games, by comparison, 90% or more of the money that comes in is returned to the players as prize money. Of course, state lotteries should be viewed as a form of voluntary taxation, not simply as games. The profits from lotteries are typically used for education, health care, and other essential services. A discussion of the value and costs of lotteries from a political and social point of view (as opposed to a mathematical one) is beyond the scope of this project.
Bonus Numbers
Many state lotteries now augment the basic \((N, n)\), format with a bonus number. The bonus number \(T\) is selected from a specified set of integers, in addition to the combination \(\bs{X}\), selected as before. The player likewise picks a bonus number \(s\), in addition to a combination \(\bs{y}\). The player's prize then depends on the number of catches \(U\) between \(\bs{X}\) and \(\bs{y}\), as before, and in addition on whether the player's bonus number \(s\) matches the random bonus number \(T\) chosen by the house. We will let \(I\) denote the indicator variable of this latter event. Thus, our interest now is in the joint distribution of \((I, U)\).
In one common format, the bonus number \(T\) is selected at random from the set of integers \(\{1, 2, \ldots, M\}\), independently of the combination \(\bs{X}\) of size \(n\) chosen from \(\{1, 2, \ldots, N\}\). Usually \(M \lt N\). Note that with this format, the game is essentially two independent lotteries, one in the \((N, n)\), format and the other in the \((M, 1)\), format.
Explicitly compute the joint probability density function of \((I, U)\) for the \((47, 5)\) lottery with independent bonus number from 1 to 27. This format is used in the California lottery, among others.
Answer
Joint distribution of \((I, U)\)
\(\P(I = i, U = k)\) | \(i = 0\) | 1 |
---|---|---|
\(k = 0\) | 0.5340250022 | 0.0205394232 |
1 | 0.3513322383 | 0.0135127784 |
2 | 0.0720681514 | 0.0027718520 |
3 | 0.0054051114 | 0.0002078889 |
4 | 0.0001318320 | 0.0000050705 |
5 | 0.0000006278 | 0.0000000241 |
Explicitly compute the joint probability density function of \((I, U)\) for the \((49, 5)\) lottery with independent bonus number from 1 to 42. This format is used in the Powerball lottery, among others.
Answer
Joint distribution of \((I, U)\)
\(\P(I = i, U = k)\) | \(i = 0\) | 1 |
---|---|---|
\(k = 0\) | 0.5559597053 | 0.0135599928 |
1 | 0.3474748158 | 0.0084749955 |
2 | 0.0677999641 | 0.0016536577 |
3 | 0.0048428546 | 0.0001181184 |
4 | 0.0001126245 | 0.0000027469 |
5 | 0.0000005119 | 0.0000000125 |
In another format, the bonus number \(T\) is chosen from 1 to \(N\), and is distinct from the numbers in the combination \(\bs{X}\). To model this game, we assume that \(T\) is uniformly distributed on \(\{1, 2, \ldots, N\}\), and given \(T = t\), \(\bs{X}\) is uniformly distributed on the set of combinations of size \(n\) chosen from \(\{1, 2, \ldots, N\} \setminus \{t\}\). For this format, the joint probability density function is harder to compute.
The probability density function of \((I, U)\) is given by \begin{align} \P(I = 1, U = k) & = \frac{\binom{n}{k} \binom{N - 1 - n}{n - k}}{N \binom{N - 1}{n}}, \quad k \in \{0, 1, \ldots, n\} \\ \P(I = 0, U = k) & = (N - n + 1) \frac{\binom{n}{k} \binom{N - 1 - n}{n - k}}{N \binom{N - 1}{n}} + n \frac{\binom{n - 1}{k} \binom{N - n}{n - k}}{N \binom{N - 1}{n}}, \quad k \in \{0, 1, \ldots, n\} \end{align}
Proof
The second equation is obtained by conditioning on whether \(T \in \{y_1, y_2, \ldots, y_n\}\).
Explicitly compute the joint probability density function of \((I, U)\) for the \((47, 7)\) lottery with bonus number chosen as described above. This format is used in the Super 7 Canada lottery, among others.
Keno
Keno is a lottery game played in casinos. For a fixed \(N\) (usually 80) and \(n\) (usually 20), the player can play a range of basic \((N, n, m)\) games, as described in the first subsection. Typically, \(m\) ranges from 1 to 15, and the payoff depends on \(m\) and the number of catches \(U\). In this section, you will compute the density function, mean, and standard deviation of the random payoff, based on a unit bet, for a typical keno game with \(N = 80\), \(n = 20\), and \(m \in \{1, 2, \ldots, 15\}\). The payoff tables are based on the keno game at the Tropicana casino in Atlantic City, New Jersey.
Recall that the probability density function of the number of catches \(U\) above , is given by \[ \P(U = k) = \frac{\binom{m}{k} \binom{80 - m}{20 - k}}{\binom{80}{20}}, \quad k \in \{0, 1, \ldots, m\} \]
The payoff table for \(m = 1\) is given below. Compute the probability density function, mean, and standard deviation of the payoff.
Catches | 0 | 1 |
---|---|---|
Payoff | 0 | 3 |
Answer
Pick \(m = 1\), \(\E(V) = 0.75\), \(\sd(V) = 1.299038106\)
\(v\) | \(\P(V = v)\) |
---|---|
0 | 0.75 |
3 | 0.25 |
The payoff table for \(m = 2\) is given below. Compute the probability density function, mean, and standard deviation of the payoff.
Catches | 0 | 1 | 2 |
---|---|---|---|
Payoff | 0 | 0 | 12 |
Answer
Pick \(m = 2\), \(E(V) = 0.7353943525\), \(\sd(V) = 5.025285956\)
\(v\) | \(\P(V = v)\) |
---|---|
12 | 0.0601265822 |
The payoff table for \(m = 3\) is given below. Compute the probability density function, mean, and standard deviation of the payoff.
Catches | 0 | 1 | 2 | 3 |
---|---|---|---|---|
Payoff | 0 | 0 | 1 | 43 |
Answer
Pick \(m = 3\), \(\E(V) = 0.7353943525\), \(\sd(V) = 5.025285956\)
\(v\) | \(\P(V = v)\) |
---|---|
0 | 0.8473709834 |
1 | 0.1387536514 |
43 | 0.0138753651 |
The payoff table for \(m = 4\) is given below. Compute the probability density function, mean, and standard deviation of the payoff.
Catches | 0 | 1 | 2 | 3 | 4 |
---|---|---|---|---|---|
Payoff | 0 | 0 | 1 | 3 | 130 |
Answer
Pick \(m = 4\), \(\E(V) = 0.7406201394\), \(\sd(V) = 7.198935911\)
\(v\) | \(\P(V = v)\) |
---|---|
0 | 0.7410532505 |
1 | 0.2126354658 |
3 | 0.0432478914 |
130 | 0.0030633923 |
The payoff table for \(m = 5\) is given below. Compute the probability density function, mean, and standard deviation of the payoff.
Catches | 0 | 1 | 2 | 3 | 4 | 5 |
---|---|---|---|---|---|---|
Payoff | 0 | 0 | 0 | 1 | 10 | 800 |
Answer
Pick \(m = 5\), \(\E(V) = 0.7207981892\), \(\sd(V) = 20.33532453\)
\(v\) | \(\P(V = v)\) |
---|---|
0 | 0.9033276850 |
1 | 0.0839350523 |
10 | 0.0120923380 |
800 | 0.0006449247 |
The payoff table for \(m = 6\) is given below. Compute the probability density function, mean, and standard deviation of the payoff.
Catches | 0 | 1 | 2 | 3 | 4 | 5 | 6 |
---|---|---|---|---|---|---|---|
Payoff | 0 | 0 | 0 | 1 | 4 | 95 | 1500 |
Answer
Pick \(m = 6\), \(\E(V) = 0.7315342885\), \(\sd(V) = 17.83831647\)
\(v\) | \(\P(V = v)\) |
---|---|
0 | 0.8384179112 |
1 | 0.1298195475 |
4 | 0.0285379178 |
95 | 0.0030956385 |
1500 | 0.0001289849 |
The payoff table for \(m = 7\) is given below. Compute the probability density function, mean, and standard deviation of the payoff.
Catches | 0 | 1 | 2 | 3 | 4 | 5 | 6 | 7 |
---|---|---|---|---|---|---|---|---|
Payoff | 0 | 0 | 0 | 0 | 1 | 25 | 350 | 8000 |
Answer
Pick \(m = 7\), \(\E(V) = 0.7196008747\), \(\sd(V) = 40.69860455\)
\(v\) | \(\P(V = v)\) |
---|---|
0 | 0.9384140492 |
1 | 0.0521909668 |
25 | 0.0086385048 |
350 | 0.0007320767 |
8000 | 0.0000244026 |
The payoff table for \(m = 8\) is given below. Compute the probability density function, mean, and standard deviation of the payoff.
Catches | 0 | 1 | 2 | 3 | 4 | 5 | 6 | 7 | 8 |
---|---|---|---|---|---|---|---|---|---|
Payoff | 0 | 0 | 0 | 0 | 0 | 9 | 90 | 1500 | 25,000 |
Answer
Pick \(m = 8\), \(\E(V) = 0.7270517606\), \(\sd(V) = 55.64771986\)
\(v\) | \(\P(V = v)\) |
---|---|
0 | 0.9791658999 |
9 | 0.0183025856 |
90 | 0.0023667137 |
1500 | 0.0001604552 |
25,000 | 0.0000043457 |
The payoff table for \(m = 9\) is given below. Compute the probability density function, mean, and standard deviation of the payoff.
Catches | 0 | 1 | 2 | 3 | 4 | 5 | 6 | 7 | 8 | 9 |
---|---|---|---|---|---|---|---|---|---|---|
Payoff | 0 | 0 | 0 | 0 | 0 | 4 | 50 | 280 | 4000 | 50,000 |
Answer
Pick \(m = 9\), \(\E(V) = 0.7270517606\), \(\sd(V) = 55.64771986\)
\(v\) | \(\P(V = v)\) |
---|---|
0 | 0.9791658999 |
9 | 0.0183025856 |
90 | 0.0023667137 |
1500 | 0.0001604552 |
25,000 | 0.0000043457 |
The payoff table for \(m = 10\) is given below. Compute the probability density function, mean, and standard deviation of the payoff.
Catches | 0 | 1 | 2 | 3 | 4 | 5 | 6 | 7 | 8 | 9 | 10 |
---|---|---|---|---|---|---|---|---|---|---|---|
Payoff | 0 | 0 | 0 | 0 | 0 | 1 | 22 | 150 | 1000 | 5000 | 100,000 |
Answer
Pick \(m = 10\), \(\E(V) = 0.7228896221\), \(\sd(V) = 38.10367609\)
\(v\) | \(\P(V = v)\) |
---|---|
0 | 0.9353401224 |
1 | 0.0514276877 |
22 | 0.0114793946 |
150 | 0.0016111431 |
1000 | 0.0001354194 |
5000 | 0.0000061206 |
100,000 | 0.0000001122 |
The payoff table for \(m = 11\) is given below. Compute the probability density function, mean, and standard deviation of the payoff.
Catches | 0 | 1 | 2 | 3 | 4 | 5 | 6 | 7 | 8 | 9 | 10 | 11 |
---|---|---|---|---|---|---|---|---|---|---|---|---|
Payoff | 0 | 0 | 0 | 0 | 0 | 0 | 8 | 80 | 400 | 2500 | 25,000 | 100,000 |
Answer
Pick \(m = 11\), \(\E(V) = 0.7138083347\), \(\sd(V) = 32.99373346\)
\(v\) | \(\P(V = v)\) |
---|---|
0 | 0.9757475913 |
8 | 0.0202037345 |
80 | 0.0036078097 |
400 | 0.0004114169 |
2500 | 0.0000283736 |
25,000 | 0.0000010580 |
100,000 | 0.0000000160 |
The payoff table for \(m = 12\) is given below. Compute the probability density function, mean, and standard deviation of the payoff.
Catches | 0 | 1 | 2 | 3 | 4 | 5 | 6 | 7 | 8 | 9 | 10 | 11 | 12 |
---|---|---|---|---|---|---|---|---|---|---|---|---|---|
Payoff | 0 | 0 | 0 | 0 | 0 | 0 | 5 | 32 | 200 | 1000 | 5000 | 25,000 | 100,000 |
Answer
Pick \(m = 12\), \(\E(V) = 0.7167721544\), \(\sd(V) = 20.12030014\)
\(v\) | \(\P(V = v)\) |
---|---|
0 | 0.9596431653 |
5 | 0.0322088520 |
32 | 0.0070273859 |
200 | 0.0010195984 |
1000 | 0.0000954010 |
5000 | 0.0000054280 |
25,000 | 0.0000001673 |
100,000 | 0.0000000021 |
The payoff table for \(m = 13\) is given below. Compute the probability density function, mean, and standard deviation of the payoff.
Catches | 0 | 1 | 2 | 3 | 4 | 5 | 6 | 7 | 8 | 9 | 10 | 11 | 12 | 13 |
---|---|---|---|---|---|---|---|---|---|---|---|---|---|---|
Payoff | 1 | 0 | 0 | 0 | 0 | 0 | 1 | 20 | 80 | 600 | 3500 | 10,000 | 50,000 | 100,000 |
Proof
Pick \(m = 13\), \(\E(V) = 0.7216651326\), \(\sd(V) = 22.68311303\)
\(v\) | \(\P(V = v)\) |
---|---|
0 | 0.9213238456 |
1 | 0.0638969375 |
20 | 0.0123151493 |
80 | 0.0021831401 |
600 | 0.0002598976 |
3500 | 0.0000200623 |
10,000 | 0.0000009434 |
50,000 | 0.0000000240 |
100,000 | 0.0000000002 |
The payoff table for \(m = 14\) is given below. Compute the probability density function, mean, and standard deviation of the payoff.
Catches | 0 | 1 | 2 | 3 | 4 | 5 | 6 | 7 | 8 | 9 | 10 | 11 | 12 | 13 | 14 |
---|---|---|---|---|---|---|---|---|---|---|---|---|---|---|---|
Payoff | 1 | 0 | 0 | 0 | 0 | 0 | 1 | 9 | 42 | 310 | 1100 | 8000 | 25,000 | 50,000 | 100,000 |
Answer
Pick \(m = 14\), \(\E(V) = 0.7194160496\), \(\sd(V) = 21.98977077\)
\(v\) | \(\P(V = v)\) |
---|---|
0 | 0.898036333063 |
1 | 0.077258807301 |
9 | 0.019851285448 |
42 | 0.004181636518 |
310 | 0.000608238039 |
1100 | 0.000059737665 |
8000 | 0.000003811015 |
25,000 | 0.000000147841 |
50,000 | 0.000000003084 |
100,000 | 0.000000000026 |
The payoff table for \(m = 15\) is given below. Compute the probability density function, mean, and standard deviation of the payoff.
Catches | 0 | 1 | 2 | 3 | 4 | 5 | 6 | 7 | 8 | 9 | 10 | 11 | 12 | 13 | 14 | 15 |
---|---|---|---|---|---|---|---|---|---|---|---|---|---|---|---|---|
Payoff | 1 | 0 | 0 | 0 | 0 | 0 | 0 | 10 | 25 | 100 | 300 | 2800 | 25,000 | 50,000 | 100,000 | 100,000 |
Answer
Pick \(m = 15\), \(\E(V) = 0.7144017020\), \(\sd(V) = 24.31901706\)
\(v\) | \(\P(V = v)\) |
---|---|
0 | 0.95333046038902 |
1 | 0.00801614417729 |
10 | 0.02988971956684 |
25 | 0.00733144064847 |
100 | 0.00126716258122 |
300 | 0.00015205950975 |
2800 | 0.00001234249267 |
25,000 | 0.00000064960488 |
50,000 | 0.00000002067708 |
100,000 | 0.00000000035046 |
100,000 | 0.00000000000234 |
In the exercises above, you should have noticed that the expected payoff on a unit bet varies from about 0.71 to 0.75, so the expected profit (for the gambler) varies from about \(-0.25\) to \(-0.29\). This is quite bad for the gambler playing a casino game, but as always, the lure of a very high payoff on a small bet for an extremely rare event overrides the expected value analysis for most players.
With \(m = 15\), show that the top 4 prizes (25,000, 50,000, 100,000, 100,000) contribute only about 0.017 (less than 2 cents) to the total expected value of about 0.714.
On the other hand, the standard deviation of the payoff varies quite a bit, from about 1 to about 55.
Although the game is highly unfavorable for each \(m\), with expected value that is nearly constant, which do you think is better for the gambler—a format with high standard deviation or one with low standard deviation?