14.7: Practice on Anxiety and Depression
- Page ID
- 18126
\( \newcommand{\vecs}[1]{\overset { \scriptstyle \rightharpoonup} {\mathbf{#1}} } \)
\( \newcommand{\vecd}[1]{\overset{-\!-\!\rightharpoonup}{\vphantom{a}\smash {#1}}} \)
\( \newcommand{\id}{\mathrm{id}}\) \( \newcommand{\Span}{\mathrm{span}}\)
( \newcommand{\kernel}{\mathrm{null}\,}\) \( \newcommand{\range}{\mathrm{range}\,}\)
\( \newcommand{\RealPart}{\mathrm{Re}}\) \( \newcommand{\ImaginaryPart}{\mathrm{Im}}\)
\( \newcommand{\Argument}{\mathrm{Arg}}\) \( \newcommand{\norm}[1]{\| #1 \|}\)
\( \newcommand{\inner}[2]{\langle #1, #2 \rangle}\)
\( \newcommand{\Span}{\mathrm{span}}\)
\( \newcommand{\id}{\mathrm{id}}\)
\( \newcommand{\Span}{\mathrm{span}}\)
\( \newcommand{\kernel}{\mathrm{null}\,}\)
\( \newcommand{\range}{\mathrm{range}\,}\)
\( \newcommand{\RealPart}{\mathrm{Re}}\)
\( \newcommand{\ImaginaryPart}{\mathrm{Im}}\)
\( \newcommand{\Argument}{\mathrm{Arg}}\)
\( \newcommand{\norm}[1]{\| #1 \|}\)
\( \newcommand{\inner}[2]{\langle #1, #2 \rangle}\)
\( \newcommand{\Span}{\mathrm{span}}\) \( \newcommand{\AA}{\unicode[.8,0]{x212B}}\)
\( \newcommand{\vectorA}[1]{\vec{#1}} % arrow\)
\( \newcommand{\vectorAt}[1]{\vec{\text{#1}}} % arrow\)
\( \newcommand{\vectorB}[1]{\overset { \scriptstyle \rightharpoonup} {\mathbf{#1}} } \)
\( \newcommand{\vectorC}[1]{\textbf{#1}} \)
\( \newcommand{\vectorD}[1]{\overrightarrow{#1}} \)
\( \newcommand{\vectorDt}[1]{\overrightarrow{\text{#1}}} \)
\( \newcommand{\vectE}[1]{\overset{-\!-\!\rightharpoonup}{\vphantom{a}\smash{\mathbf {#1}}}} \)
\( \newcommand{\vecs}[1]{\overset { \scriptstyle \rightharpoonup} {\mathbf{#1}} } \)
\( \newcommand{\vecd}[1]{\overset{-\!-\!\rightharpoonup}{\vphantom{a}\smash {#1}}} \)
\(\newcommand{\avec}{\mathbf a}\) \(\newcommand{\bvec}{\mathbf b}\) \(\newcommand{\cvec}{\mathbf c}\) \(\newcommand{\dvec}{\mathbf d}\) \(\newcommand{\dtil}{\widetilde{\mathbf d}}\) \(\newcommand{\evec}{\mathbf e}\) \(\newcommand{\fvec}{\mathbf f}\) \(\newcommand{\nvec}{\mathbf n}\) \(\newcommand{\pvec}{\mathbf p}\) \(\newcommand{\qvec}{\mathbf q}\) \(\newcommand{\svec}{\mathbf s}\) \(\newcommand{\tvec}{\mathbf t}\) \(\newcommand{\uvec}{\mathbf u}\) \(\newcommand{\vvec}{\mathbf v}\) \(\newcommand{\wvec}{\mathbf w}\) \(\newcommand{\xvec}{\mathbf x}\) \(\newcommand{\yvec}{\mathbf y}\) \(\newcommand{\zvec}{\mathbf z}\) \(\newcommand{\rvec}{\mathbf r}\) \(\newcommand{\mvec}{\mathbf m}\) \(\newcommand{\zerovec}{\mathbf 0}\) \(\newcommand{\onevec}{\mathbf 1}\) \(\newcommand{\real}{\mathbb R}\) \(\newcommand{\twovec}[2]{\left[\begin{array}{r}#1 \\ #2 \end{array}\right]}\) \(\newcommand{\ctwovec}[2]{\left[\begin{array}{c}#1 \\ #2 \end{array}\right]}\) \(\newcommand{\threevec}[3]{\left[\begin{array}{r}#1 \\ #2 \\ #3 \end{array}\right]}\) \(\newcommand{\cthreevec}[3]{\left[\begin{array}{c}#1 \\ #2 \\ #3 \end{array}\right]}\) \(\newcommand{\fourvec}[4]{\left[\begin{array}{r}#1 \\ #2 \\ #3 \\ #4 \end{array}\right]}\) \(\newcommand{\cfourvec}[4]{\left[\begin{array}{c}#1 \\ #2 \\ #3 \\ #4 \end{array}\right]}\) \(\newcommand{\fivevec}[5]{\left[\begin{array}{r}#1 \\ #2 \\ #3 \\ #4 \\ #5 \\ \end{array}\right]}\) \(\newcommand{\cfivevec}[5]{\left[\begin{array}{c}#1 \\ #2 \\ #3 \\ #4 \\ #5 \\ \end{array}\right]}\) \(\newcommand{\mattwo}[4]{\left[\begin{array}{rr}#1 \amp #2 \\ #3 \amp #4 \\ \end{array}\right]}\) \(\newcommand{\laspan}[1]{\text{Span}\{#1\}}\) \(\newcommand{\bcal}{\cal B}\) \(\newcommand{\ccal}{\cal C}\) \(\newcommand{\scal}{\cal S}\) \(\newcommand{\wcal}{\cal W}\) \(\newcommand{\ecal}{\cal E}\) \(\newcommand{\coords}[2]{\left\{#1\right\}_{#2}}\) \(\newcommand{\gray}[1]{\color{gray}{#1}}\) \(\newcommand{\lgray}[1]{\color{lightgray}{#1}}\) \(\newcommand{\rank}{\operatorname{rank}}\) \(\newcommand{\row}{\text{Row}}\) \(\newcommand{\col}{\text{Col}}\) \(\renewcommand{\row}{\text{Row}}\) \(\newcommand{\nul}{\text{Nul}}\) \(\newcommand{\var}{\text{Var}}\) \(\newcommand{\corr}{\text{corr}}\) \(\newcommand{\len}[1]{\left|#1\right|}\) \(\newcommand{\bbar}{\overline{\bvec}}\) \(\newcommand{\bhat}{\widehat{\bvec}}\) \(\newcommand{\bperp}{\bvec^\perp}\) \(\newcommand{\xhat}{\widehat{\xvec}}\) \(\newcommand{\vhat}{\widehat{\vvec}}\) \(\newcommand{\uhat}{\widehat{\uvec}}\) \(\newcommand{\what}{\widehat{\wvec}}\) \(\newcommand{\Sighat}{\widehat{\Sigma}}\) \(\newcommand{\lt}{<}\) \(\newcommand{\gt}{>}\) \(\newcommand{\amp}{&}\) \(\definecolor{fillinmathshade}{gray}{0.9}\)
Our first example will focus on variables related to health: mental health. Our hypothesis testing procedure follows the same four-step process as before, starting with our research and null hypotheses.
Scenario
Anxiety and depression are often reported to be highly linked (or “co-morbid”). We will test whether higher scores on an anxiety scale are related to higher scales on a depression scale, lower scores on the depression scale, or not related to scores on a depression scale (meaning that the two variables do not vary together). We have scores on an anxiety scale and a depression scale for a group of 10 people. Because both scales are measured by numbers, this means that we have two different quantitative variables for the same people; perfect for a correlational statistical analysis!
Step 1: State the Hypotheses
For a research hypothesis for correlation analyses, we are predicting that there will be a positive linear relationship or a negative linear relationship. A positive linear relationship means that the two variables vary in the same direction (when one goes up, the other also goes up), while a negative linear relationship means that the two variables vary in opposite directions (when one goes up, the other goes down). We keep the word "linear" in the hypotheses to keep reminding ourselves that Pearson's r can only detect linear relationships. If we expect something else, then Pearson's r is not the correct analysis.
Okay, let's start with a research hypothesis. If the two variables are co-morbid, do you think that the would vary in the same direction or in opposite directions? Use that to determine your research hypothesis.
Example \(\PageIndex{1}\)
What is a research hypothesis for this scenario?
Solution
The research hypothesis should be: There is positive linear relationship between anxiety scores and depression scores.
Look back at the research hypothesis above in Example \(\PageIndex{1}\). Does it include the names of both variables (what was measured)? Yes. Does it state whether the relationship will be positive or negative? Yes. Does it include the phrase "linear relationship"? Yes. Yay, we have all of the important parts of a research hypothesis! Does it say anything about means? No, which is fine; correlations aren't comparing means. Although knowing the means and standard deviations of each variable might be interesting, that descriptive information is not really necessary to test whether the two variables are linearly related.
Let's move on to the null hypothesis. What do you think that will look like?
Example \(\PageIndex{2}\)
What is a null hypothesis for this scenario?
Solution
The null hypothesis should be: There is no linear relationship between anxiety scores and depression scores..
Notice that the variables are included again, and the word "linear".
Step 2: Find the Critical Values
The critical values for correlations come from the Table of Critical Values of r , which looks very similar to the \(t\)-table. Just like our \(t\)-table, the row is determined by our degrees of freedom. For correlations, we have \(N – 2\) degrees of freedom, rather than \(N – 1\) (why this is the case is not important). For our example, we have 10 people.
We were not given any information about the level of significance at which we should test our hypothesis, so we will assume \(α = 0.05\). From the table, we can see that at p = .05 level, the critical value of rCritical = 0.576. Thus, if our calculated correlation is greater than 0.576, it will be statistically significant and we would reject the null hypothesis. This is a rather high bar (remember, the guideline for a strong relation is \(r\) = 0.50); this is because we have so few people. Larger samples make it easier to find significant relations.
Step 3: Calculate the Test Statistic
We have laid out our hypotheses and the criteria we will use to assess them, so now we can move on to our test statistic. Before we do that, we should first create a scatterplot of the data to make sure that the most likely form of our relation is in fact linear. Figure \(\PageIndex{2}\) below shows our data plotted out. Dr. MO is not convinced that there's a strong linear relationship, but there doesn't seem to be a strong curvilinear relationship, either. Dr. Foster thinks that the dots are, in fact, linearly related, so Pearson’s \(r\) is appropriate.
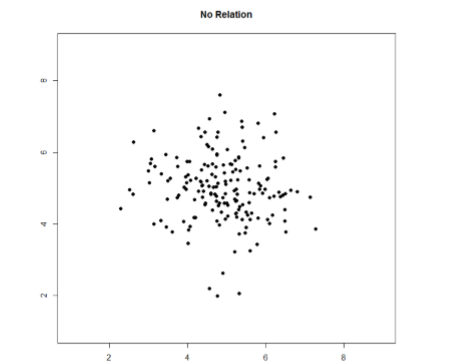
The data we gather from our participants is shown in Table \(\PageIndex{1}\). The sum for each variable is also provided so that it's easy to calculate the mean.
Depression Scores | Anxiety Scores |
---|---|
2.81 | 3.54 |
1.96 | 3.05 |
3.43 | 3.81 |
3.40 | 3.43 |
4.71 | 4.03 |
1.80 | 3.59 |
4.27 | 4.17 |
3.68 | 3.46 |
2.44 | 3.19 |
3.13 | 4.12 |
\(\sum \) = 31.63 | \(\sum \) = 36.39 |
It's easiest to calculate the formula that we're using for correlations by using a table to find the difference from the mean for each participant and multiplying them. Let's start by finding the means.
Exercise \(\PageIndex{1}\)
What is the average depression score? What is the average anxiety score?
- Answer
-
\[ \displaystyle \bar{X_D} = \dfrac{\sum X}{N} = \dfrac{31.63}{10} = 3.163 = 3.16 \nonumber \]
\[ \displaystyle \bar{X_A} = \dfrac{\sum X}{N} = \dfrac{36.39}{10} = 3.639 =3.64 \nonumber \]
Now that you've done that, let's put the means and the standard deviations in Table \(\PageIndex{2}\). We can learn a littlebit about the two variables from this information alone. It looks like the participants might be more anxious than they are depressed. This conclusion is very tentative, however, for two reasons. First, we didn't conduct a t-test to see if the means are statistically significantly different from each other. Second, we don't actually know that the two scales have the same range! Maybe the Depression Scale ranges from 1-5, and the Anxiety Scale ranges from 0-10; we don't really have enough information to make any final determines about the means with only this table. However, it also appears that there is more variability in the Depression Scale than the Anxiety Scale, but again, we don't know that for sure unless we do some other statistical analyses.
N | Mean | Standard Deviation | |
---|---|---|---|
Depression Scale | 10 | 3.16 | 0.94 |
Anxiety Scale | 10 | 3.64 | 0.38 |
But since we're trying to conduct a correlational analysis:
\[ r= \cfrac{ \left( \cfrac{\sum ((x_{Each} - \bar{X_x})*(y_{Each} - \bar{X_y}) ) }{(N-1)}\right) } {(s_x * s_y)} \nonumber \]
let's get going by filling in Table \(\PageIndex{3}\). Because we were provided the standard deviations, we do not need columns to square the differences from the means so those columns are not included in Table \(\PageIndex{3}\).
Example \(\PageIndex{3}\)
Fill in Table \(\PageIndex{3}\). Keep the negative signs!
Depression Score | Depression Difference (Depression Score Minus Depression Mean) | Anxiety Score | Anxiety Difference (Anxiety Score Minus Anxiety Mean) | Depression Difference Times Anxiety Difference |
---|---|---|---|---|
2.81 | 3.54 | |||
1.96 | 3.05 | |||
3.43 | 3.81 | |||
3.40 | 3.43 | |||
4.71 | 4.03 | |||
1.80 | 3.59 | |||
4.27 | 4.17 | |||
3.68 | 3.46 | |||
2.44 | 3.19 | |||
3.13 | 4.12 | |||
\(\sum \) = 31.63 | \(\sum \) = ? | \(\sum \) = 36.39 | \(\sum \) = ? | \(\sum \) = ? |
Solution
Now it's Table \(\PageIndex{4}\):
Depression Score | Depression Difference (Depression Score Minus Depression Mean) | Anxiety Score | Anxiety Difference (Anxiety Score Minus Anxiety Mean) | Depression Difference Times Anxiety Difference |
---|---|---|---|---|
2.81 | -0.35 | 3.54 | -0.10 | 0.04 |
1.96 | -1.20 | 3.05 | -0.59 | 0.71 |
3.43 | 0.27 | 3.81 | 0.17 | 0.05 |
3.40 | 0.24 | 3.43 | -0.21 | -0.05 |
4.71 | 1.55 | 4.03 | 0.39 | 0.60 |
1.80 | -1.36 | 3.59 | -0.05 | 0.07 |
4.27 | 1.11 | 4.17 | 0.53 | 0.59 |
3.68 | 0.52 | 3.46 | -0.18 | -0.09 |
2.44 | -0.72 | 3.19 | -0.45 | 0.32 |
3.13 | -0.03 | 4.12 | 0.48 | -0.01 |
\(\sum \) = 31.63 | \(\sum \) = 0.03 | \(\sum \) = 36.39 | \(\sum \) = -0.01 | \(\sum \) = 2.22 |
The bottom row is the sum of each column. The difference scores for Depression sum to 0.03 and the differences scores fo Anxiety sum to -0.1; both of these are very close to 0 so, given rounding error, everything looks right so far. If you had a spreadsheet conduct all of your computations, your sums of the differences will be slightly different. However, it all sorta washes out because the final sum of the products is 2.22 no matter how many decimals you save.
Okay, let's look at the formula again to see what information we might still need:
\[ r= \cfrac{ \left( \cfrac{\sum ((x_{Each} - \bar{X_x})*(y_{Each} - \bar{X_y}) ) }{(N-1)}\right) } {(s_x * s_y)} \nonumber \]
Hey, it looks like we have all of the numbers that we need! But let's replace the "x" and "y" with D for Depression and A for Anxiety. That gives us:
\[ r= \cfrac{ \left( \cfrac{\sum ((D_{Each} - \bar{X_D})*(A_{Each} - \bar{X_A}) ) }{(N-1)}\right) } {(s_D * s_A)} \nonumber \]
Example \(\PageIndex{4}\)
Use the information provided and that we've calculated to complete the correlational analysis:
\[ r= \cfrac{ \left( \cfrac{\sum ((D_{Each} - \bar{X_D})*(A_{Each} - \bar{X_A}) ) }{(N-1)}\right) } {(s_D * s_A)} \nonumber \]
Solution
\[ r_{Filled In}= \cfrac{ \left( \cfrac{2.22 }{(10-1)}\right) } {(0.94 * 0.38 )} \nonumber \]
It's pretty crazy, but all of that mess at the very top of the formula (the numerator of the numerator) is what we did in the table, so it boils down to 2.22. After that, it's pretty easy!
\[ r_{Parentheses}= \cfrac{ \left( \cfrac{2.22 }{9}\right) } {0.36} \nonumber \]
\[ r_{Divide}= \dfrac{ 0.25 } {0.36} \nonumber \]
\[ r = 0.69 \nonumber \].
If you use a spreadsheet to keep all of the decimal points, you might end up with r=0.68 or if you round differently you could get r=0.70. Since we're learning the process right now, these are all close enough.
So our calculated correlation between anxiety and depression is \(r=0.69\), which is a strong, positive correlation. Now we need to compare it to our critical value to see if it is also statistically significant.
Step 4: Make a Decision
Our critical value was rCritical = 0.576 and our calculated value was \(rCalc = 0.69\). Our calculated value was larger than our critical value, so we can reject the null hypothesis because this is still true:
Critical \(<\) Calculated \(=\) Reject null \(=\) There is a linear relationship. \(= p<.05 \)
Critical \(>\) Calculated \(=\) Retain null \(=\) There is not a linear relationship. \(= p>.05\)
The statistical sentence is similar to what we've been doing, so let's try that now:
Example \(\PageIndex{6}\)
What is the statistical sentence for the results for our correlational analysis?
Solution
The statistical sentence is: r(8)=0.69, p<.05
Because the Degrees of Freedom are N-2 for Pearson's r.
Okay, we're ready for the final write-up! Because our r = 0.69, a positive number, we can say that the linear relationship is positive.
Example \(\PageIndex{7}\)
Write up your conclusion. Don't forget to include the four requirements for reporting results!
Solution
"We hypothesized that there would be a positive linear relationship between depression scores (M=3.16) and anxiety scores (M=3.64). This hypothesis was supported (r(8)=0.69, p < .05). As depression scores increase, anxiety scores also increase. With our sample, depression and anxiety do appear to be co-morbid."
As we will discover in the next chapter, we can also add a sentence about predictions: "Based on these results, we can use a participant's depression score to predict their anxiety score."
Even though we are dealing with a very different type of data, our process of hypothesis testing has remained unchanged.
Let's try that again, but use the full Sum of Products table to calculate the standard deviations, too.
Contributors and Attributions
Foster et al. (University of Missouri-St. Louis, Rice University, & University of Houston, Downtown Campus)