13.1: Introduction to Factorial Designs
- Page ID
- 17397
Research Designs
Phew, we've covered a lot in the past few chapters! Each of the inferential statistical analyses that we've covered is to compare means (quantitative variable) between different groups. The qualitative variable is the two different samples or an IV with two or more levels. With t-tests, with compared two different groups (which can be considered one IV with two levels). Sometimes, those groups were unrelated (independent t-test) and sometimes they were related (dependent t-test). With ANOVAs, we compared one IV with two or more levels. Sometimes those IV levels were unrelated (Between Groups ANOVA) and sometimes they were related (Repeated Measures ANOVA, sometimes called Within-Groups ANOVA).
ANOVAs are an amazing statistical tool because they also allow is to compare means for a combination of IVs (not just IV levels). This happens in a factorial design, when each level of each IV is combined so that a set of participants experiences the combination of levels of each IV.
Let’s try an example. Let’s say that we wanted to test to see if mindset (growth versus fixed mindset) affects how long students spend on their homework.
Exercise \(\PageIndex{1}\)
What would be the IV and IV levels? What would be the DV?
- Answer
-
The IV would be Mindset, with the levels being growth mindset or fixed mindset.
The DV would be how long student spend on their homework.
As an educational researcher, I also know that outside obligations have a lot to do with how much time students can spend on their homework. To test for this, let’s add another IV to our experiment called “Job” with the levels of: Full-Time, Part-Time, None.
Now we have a factorial design! We have two IVs, Mindset and Job. Mindset has two levels and Job has three levels. We have one DV, how long student spend on homework. This means that I’ll measure how much time students spend on homework for:
- Students with growth mindset who work full-time,
- Students with growth mindset who work part-time,
- Students with growth mindset who don’t work,
- Students with fixed mindset who work full-time,
- Students with fixed mindset who work part-time, and
- Students with fixed mindset who don’t work.
This helps us understand how mindset affects homework time for students who work different hours and can give us a lot more information than a study with just one of those IVs. For example, folks with growth mindset know that success comes after putting in time and learning from their mistakes, so maybe whether you work full-time or doesn’t matter for students with growth mindset, but how much you work does affect how long students with fixed mindset spend on homework. Can you see how combining the IVs gives us more information?
Cause & Effect
Researchers often use factorial designs to understand the causal influences behind the effects they are interested in improving. Effects are the change in a measure (DV) caused by a manipulation (IV levels). You get an effect any time one IV causes a change in a DV.
Distraction Scenario
Here is an example. We will stick with this one example for a while, so pay attention… In fact, the example is about paying attention! Let’s say you wanted to measure something like paying attention. You could something like this:
- Pick a task for people to do that you can measure. That will be the dependent variable. This is the effect.
- Pick something that you think will cause differences in paying attention. For example, we know that people can get distracted easily when there are distracting things around. This is your IV. You could have two levels for your manipulation: No distraction versus distraction. This is what we think is the cause of changes in the DV.
- Measure performance in the task (DV) under the conditions (IV levels or groups).
- If your distraction manipulation changes how people perform the task, you may have successfully manipulated how well people can pay attention in your task.
1. Pick a Task (DV)
First, we pick a task. It’s called Spot the Difference. You may have played this game before. You look at two pictures side-by-side, and then you locate as many differences as you can find. Figure \(\PageIndex{1}\) is an example:
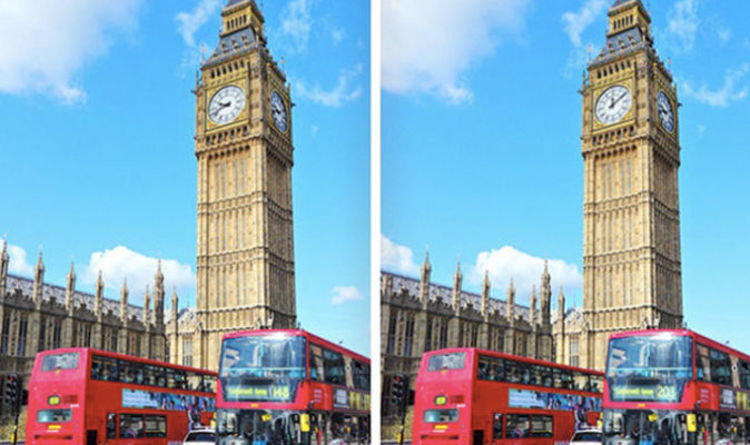
How many differences can you spot? When you look for the differences, it feels like you are doing something we would call “paying attention”. If you pay attention to the clock tower, you will see that the hands on the clock are different. Yay! One difference spotted. We could give people 30 seconds to find as many differences as they can. Then we give them another set of pictures and do it again. Every time we will measure how many differences they can spot. So, our measure of performance, our dependent variable, could be the mean number of differences spotted.
That gives us an effect on our IV. But there are no groups here, no levels. Everyone gets the same pictures and does the same thing, so we're not actually testing anything.
2. Pick a Cause (IV)
Now, let’s think about what might cause differences in how people pay attention. If people need to pay attention to spot differences, then presumably if we made it difficult to pay attention, people would spot less differences. What is a good way to distract people? I’m sure there are lots of ways to do this. How about we do the following:
- No distraction condition: People do the task with no added distractions. They sit in front of a computer, in a quiet, distraction-free room, and find as many differences as they can for each pair of pictures. This is the comparison condition, and is sometimes called a "control" because the participants experience the "nothing" version of the IV levels.
- Distraction condition: We blast super loud ambulance sounds and fire alarms and children's music while people attempt to spot differences. We also randomly turn the sounds on and off, and make them super-duper annoying and distracting. We make sure that the sounds aren’t loud enough to do any physical damage to anybody’s eardrums, but loud enough to be super distracting. If you don’t like this, we could also tickle people with a feather, or whisper silly things into their ears, or surround them by clowns, or whatever we want, it just has to be super distracting.
3. & 4. Measure Performance to Find an Effect
If our distraction manipulation is super-distracting, then what should we expect to find when we compare spot-the-difference performance between the no-distraction and distraction conditions? We should find a difference! If our manipulation works, then we should find that people find more differences when they are not distracted, and less differences when they are distracted.
Imagine that the results show that people found an average of four differences when they were distracted, and an average of 10 differences when they were not distracted. The effect of distraction is a mean of six differences found in the picture. It’s the difference between performance in the Distraction and No-Distraction conditions. In general, it is very common to use the word effect to refer to the differences caused by the IV. We manipulated distraction, it caused a difference, so we call this the “distraction effect”.
How is this related to Factorial Designs?
You might be wondering how this scenario on distractions and Spotting the Difference is related to factorial designs and ANOVAs since a t-test would be great at analyzing this data. The answer is that science is cumulative.
We have done the hard work of finding an effect of interest, in this case the distraction effect. We think this distraction effect actually measures something about your ability to pay attention. But why stop there? If we think that we found an effect of distraction, maybe we could find a way to reduce distraction to help students focus on their homework? Or help employees focus on their jobs? Or help my partner focus on the intricate plot of TV show that we're watching? Or what if we found some individual differences, meaning that some people get distracted easier than other people? You were the kind of person who had a small distraction effect (maybe you find 10 differences when you are not distracted, and 9 differences when you are distracted), that could mean you are very good at ignoring distracting things while you are paying attention. On the other hand, you could be the kind of person who had a big distraction effect (maybe you found 10 differences under no distraction, and only one difference when you were distracted); this could mean you are not very good at ignoring distracting things while you are paying attention.
Overall now, we are thinking that our effect of distraction (IV) on spotting the difference (DV) by finding the difference in performance between the two conditions might be affected by more than just being distracted. This may lead us to want to know how to make people better at ignoring distracting things. Our first stab at science found that distraction affected paying attention, but we want to expand on that finding. Maybe now we want to know what makes people worse at ignoring things? In other words, we want to find out what other IVs might affect the size of the distraction effect (make it bigger or smaller, or even flip around!).
We are going to add a second IV to our task. Note that this is not a new level to our Distraction IV, but a whole new IV. The second IV will be reward. In one condition, people will get $5 for every difference they find (so they could leave the study with lots of money if they find lots of differences). In the other condition, people will get no money, but they will still have find differences (comparison control condition).
We could run this study on it's own, comparing receiving a reward to not receiving a reward. But this will be a factorial design, so everybody will have to find differences. A quarter of the sample will find differences when they are distracted but they will get a reward for each difference that they find. A quarter of the sample will find differences whey they are distracted, but won't know anything about a reward. A quarter of the sample will look for differences without being distracted, but they will be rewarded for each difference they found. And finally, a quarter of the sample will look for differences without being distracted and will also not earn a reward. Thus, the factorial design has the folloing combinations:
- Distracted with reward
- Distracted with no reward
- Not distracted with reward
- Not distracted with no reward
Note that the DV is the same for all of the IV conditions: how many differences were spotted.
'Results
The question we are now asking is: Will the reward IV cause a change in the size of the distraction effect? We could predict that people receiving rewards will have a smaller distraction effect than people not receiving rewards.
- No-Reward condition: In the no-reward condition people played Spot the Difference when they were distracted and when they were not distracted. This is a replication of our first study. We should expect to find the same pattern of results, and that’s what we found: People found six differences when they were distracted and 10 when they were not distracted. So, there was a distraction effect of four, same as we had last time.
- Reward condition: In the reward condition people played Spot the Difference when they were distracted and when they were not distracted. Except, they got $5 every time they spotted a difference. We predicted this would cause people to pay more attention and do a better job of ignoring distracting things. This is what happened. People found nine differences when they were distracted and 11 when they were not distracted. So, there was a distraction effect of two.
We conclude that reward can manipulate the distraction effect. When there was no reward, the size of the distraction effect was four. When there was reward, the size of the distraction effect was two. So, the reward manipulation changed the size of the distraction effect by two (4-2=2).
Why Factorial Designs?
Factorial designs are so useful because they allow researchers to find out what kinds of variables can cause changes in the effects they measure. We measured the distraction effect, then we found that reward causes changes in the distraction effect. If we were trying to understand how paying attention works, we would then need to explain how it is that reward levels could causally change how people pay attention (because science is cumulative). We would have some evidence that reward does cause change in paying attention, and we would have to come up with some explanations, and then run more experiments to test whether those explanations hold water.
That’s the basic idea of factorial designs, but of course it gets more complex pretty quickly. To help researchers quickly identify what's going on in a factorial design, we use specific notation. It's a lot of new information for students, so pay attention!