4.4: If at First You Don't Succeed, Try, Try Again
- Page ID
- 27626
\( \newcommand{\vecs}[1]{\overset { \scriptstyle \rightharpoonup} {\mathbf{#1}} } \)
\( \newcommand{\vecd}[1]{\overset{-\!-\!\rightharpoonup}{\vphantom{a}\smash {#1}}} \)
\( \newcommand{\id}{\mathrm{id}}\) \( \newcommand{\Span}{\mathrm{span}}\)
( \newcommand{\kernel}{\mathrm{null}\,}\) \( \newcommand{\range}{\mathrm{range}\,}\)
\( \newcommand{\RealPart}{\mathrm{Re}}\) \( \newcommand{\ImaginaryPart}{\mathrm{Im}}\)
\( \newcommand{\Argument}{\mathrm{Arg}}\) \( \newcommand{\norm}[1]{\| #1 \|}\)
\( \newcommand{\inner}[2]{\langle #1, #2 \rangle}\)
\( \newcommand{\Span}{\mathrm{span}}\)
\( \newcommand{\id}{\mathrm{id}}\)
\( \newcommand{\Span}{\mathrm{span}}\)
\( \newcommand{\kernel}{\mathrm{null}\,}\)
\( \newcommand{\range}{\mathrm{range}\,}\)
\( \newcommand{\RealPart}{\mathrm{Re}}\)
\( \newcommand{\ImaginaryPart}{\mathrm{Im}}\)
\( \newcommand{\Argument}{\mathrm{Arg}}\)
\( \newcommand{\norm}[1]{\| #1 \|}\)
\( \newcommand{\inner}[2]{\langle #1, #2 \rangle}\)
\( \newcommand{\Span}{\mathrm{span}}\) \( \newcommand{\AA}{\unicode[.8,0]{x212B}}\)
\( \newcommand{\vectorA}[1]{\vec{#1}} % arrow\)
\( \newcommand{\vectorAt}[1]{\vec{\text{#1}}} % arrow\)
\( \newcommand{\vectorB}[1]{\overset { \scriptstyle \rightharpoonup} {\mathbf{#1}} } \)
\( \newcommand{\vectorC}[1]{\textbf{#1}} \)
\( \newcommand{\vectorD}[1]{\overrightarrow{#1}} \)
\( \newcommand{\vectorDt}[1]{\overrightarrow{\text{#1}}} \)
\( \newcommand{\vectE}[1]{\overset{-\!-\!\rightharpoonup}{\vphantom{a}\smash{\mathbf {#1}}}} \)
\( \newcommand{\vecs}[1]{\overset { \scriptstyle \rightharpoonup} {\mathbf{#1}} } \)
\( \newcommand{\vecd}[1]{\overset{-\!-\!\rightharpoonup}{\vphantom{a}\smash {#1}}} \)
The base rate fallacy shows us that false positives are much more likely than you’d expect from a \(p<0.05\) criterion for significance. Most modern research doesn’t make one significance test, however; modern studies compare the effects of a variety of factors, seeking to find those with the most significant effects.
For example, imagine testing whether jelly beans cause acne by testing the effect of every single jelly bean color on acne:
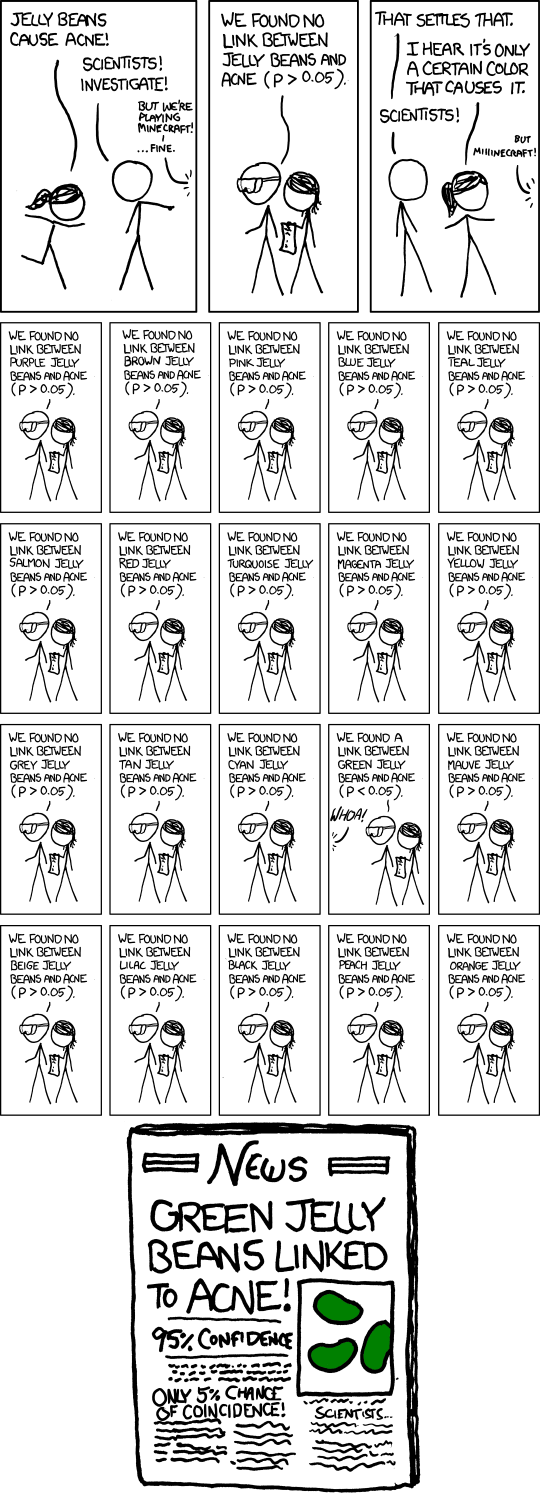
As you can see, making multiple comparisons means multiple chances for a false positive. For example, if I test \(20\) jelly bean flavors which do not cause acne at all, and look for a correlation at \(p<0.05\) significance, I have a \(64\)% chance of a false positive result.54 If I test \(45\) materials, the chance of false positive is as high as \(90\)%.
It’s easy to make multiple comparisons, and it doesn’t have to be as obvious as testing twenty potential medicines. Track the symptoms of a dozen patients for a dozen weeks and test for significant benefits during any of those weeks: bam, that’s twelve comparisons. Check for the occurrence of twenty-three potential dangerous side effects: alas, you have sinned. Send out a ten-page survey asking about nuclear power plant proximity, milk consumption, age, number of male cousins, favorite pizza topping, current sock color, and a few dozen other factors for good measure, and you’ll find that something causes cancer. Ask enough questions and it’s inevitable.
A survey of medical trials in the 1980s found that the average trial made \(30\) therapeutic comparisons. In more than half of the trials, the researchers had made so many comparisons that a false positive was highly likely, and the statistically significant results they did report were cast into doubt: they may have found a statistically significant effect, but it could just have easily been a false positive.54
There exist techniques to correct for multiple comparisons. For example, the Bonferroni correction method says that if you make \(n\) comparisons in the trial, your criterion for significance should be \(p<0.05/n\). This lowers the chances of a false positive to what you’d see from making only one comparison at \(p<0.05\). However, as you can imagine, this reduces statistical power, since you’re demanding much stronger correlations before you conclude they’re statistically significant. It’s a difficult tradeoff, and tragically few papers even consider it.